Understanding Predictive Customer Behavior Modeling in Performance Marketing
April 30, 2024
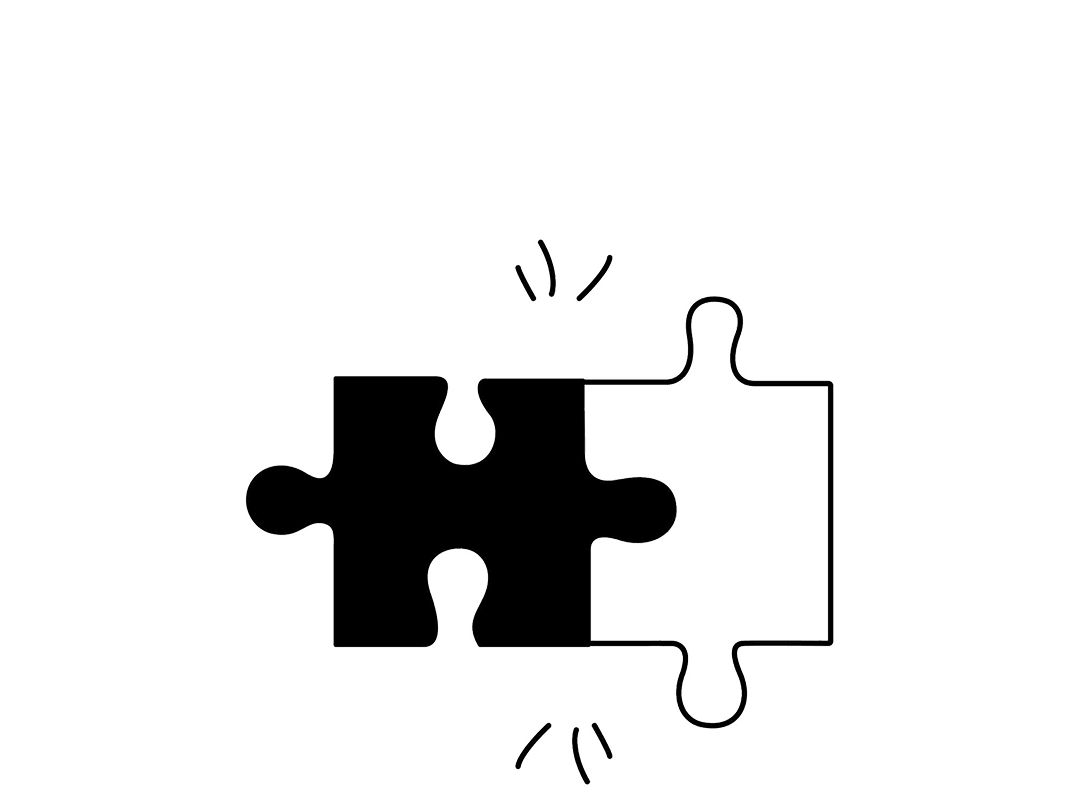
Understanding Predictive Customer Behavior Modeling in Performance Marketing
Discover how predictive customer behavior modeling using AI can revolutionise performance marketing by anticipating consumer actions, enhancing targeting strategies, and boosting ROI.
Introduction:
Predictive customer behavior modeling is a game-changing approach in performance marketing, allowing businesses to anticipate potential consumer actions and tailor their marketing strategies accordingly. By leveraging artificial intelligence (AI), companies can predict future behaviors based on historical data, leading to more effective and proactive marketing efforts.
The Role of AI in Predictive Behavior Modeling:
AI algorithms play a critical role in predictive behavior modeling by analyzing large sets of data to identify patterns and predict future actions. This capability enables marketers to understand customer propensities, such as the likelihood of purchasing a product, responding to a campaign, or churning.
Benefits of Predictive Modeling for Start-Ups:
For start-ups, predictive modeling can be particularly beneficial as it allows for more precise allocation of marketing resources, ensuring that efforts are not wasted on unlikely prospects. This strategic approach can significantly reduce costs and increase the efficiency of customer acquisition and retention strategies.
Strategies to Implement Predictive Modeling:
- Data Collection: Gather comprehensive data from various touchpoints throughout the customer journey.
- Machine Learning Models: Employ machine learning algorithms to analyze the data and develop predictions about future behaviors.
- Integration into Marketing Campaigns: Utilise these insights to craft targeted campaigns that speak directly to the predicted needs and behaviors of different customer segments.
Case Studies and Literature:
Businesses like Spotify and Target have successfully implemented predictive behavior modeling, resulting in enhanced customer experiences and increased sales (source: Business Insider). Academic resources such as the book 'Data Science for Business' by Provost and Fawcett provide foundational knowledge and practical applications for using data analytics in marketing strategies.
Additional Resources:
Journals like 'Marketing Science' and platforms such as Google Analytics and Adobe Analytics offer tools and studies that provide deeper insights into the effectiveness of predictive modeling in marketing.
Conclusion:
Predictive customer behavior modeling is transforming performance marketing by providing deep insights into future consumer actions. For startups, integrating AI-driven predictive analytics into their marketing strategies can lead to significant competitive advantages, enabling smarter, more effective campaigns that drive higher engagement and increased returns. Adopting these advanced techniques will not only save costs but also improve overall business performance through more informed decision-making.